In an online seminar, Prof. Cameron Campbell used datasets of 3 projects to illustrate research data management practices. His advises are not only applicable for historical data, but also valuable for researchers in many disciplines.
Prof. Cameron Campbell (Division of Social Science, HKUST) and the Lee-Campbell research group have been constructing datasets of Chinese historical data for over 40 years. He shared with the seminar audience the stories of three of his datasets, and gave us a lot of practical tips in curating and sharing research data. This post revisits his talk, and highlights four good practices among the many covered in the talk.
The Datasets
The datasets of the Lee-Campbell group contain quantitative data on population and families in China spanning from mid-1700’s to early 20th Century. They are transcribed from primary sources including historical archives and population registers. While Prof. Campbell’s research focuses on social stratification and inequality, the datasets have high academic value beyond his research interests. Therefore, having the data well-preserved and well-shared is a considerable contribution to scholarship. In the seminar, Prof. Campbell described the formation of 3 datasets:
China Multi-Generational Panel Dataset -Liaonin (CMGPD-LN)
- Contains 1.5 million records describing 260,000 people between 1749 and 1909; over 1,000 paternal descent groups identified through record linkage through 7 generations
- Data source: microfilmed materials in Liaoning Provincial Archives
- The dataset has been cited in nearly 100 publications by members of the research group and collaborators, as well as by others in papers that have appeared in Demography, China Economic Review, and other journals
- Dataset is available at ICPSR
China Multi-Generational Panel Dataset -Shuangcheng (CMGPD-SC)
- Contains longitudinal individual, household, and community information on the demographic and socioeconomic characteristics of a migrant population living in Shuangcheng (1866 to 1912)
- Data source: Qing population registers (260 coded and linked registers from 1866 to 1913)
- Publicly released at ICPSR
China Government Employee Database -Qing (CGED-Q)
- Contains 4,195,280 records of 326,482 Qing officials, 1830-1912. For each individual, it captures name, county of origin, examination degree, banner affiliation, bureaucratic rank, the posting and more
- Data source: Jinshenlu (缙绅录), which listed Qing civil officials. Tsinghua University Library published their collection in 2008
- Released at DataSpace@HKUST
Data Management Best Practices
Prof. Campbell outlined 6 stages of constructing datasets from primary sources:
- Acquisition
- Entry
- Documentation
- Sharing
- Public release
- Outreach
These steps can guide researchers working with historical data as well as contemporary data. He further shared some practical advises on:
Transcribing data from image sources
To ensure data consistency and quality, coders who transcribe the data from the historical image sources should be given clear instructions. Researchers should therefore minimize the judgments that coders have to make, do not ask nor allow coders to make judgment calls, or ‘correct’ obviously wrong data.
Since the data may have unanticipated applications in the future, transcribing process should be comprehensive. Code all data available in the source.
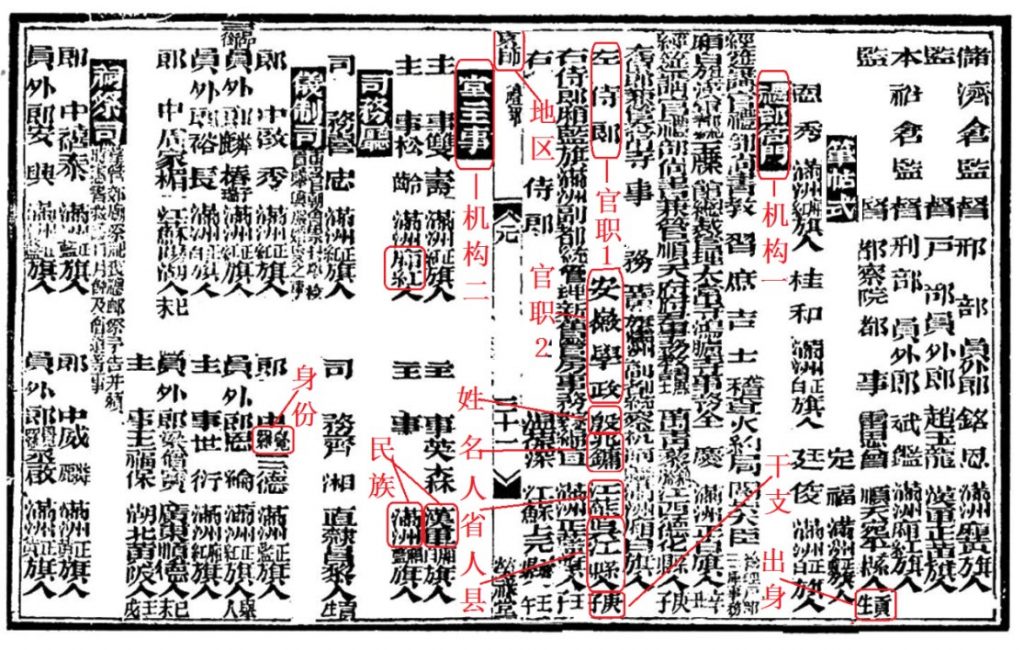
Encoding data elements from a page of Jinshenlu
Documenting ownership agreements and data decisions
Historical datasets are normally constructed from sources in libraries, archives, or in some cases private collections. When acquiring sources, researchers should play by the rules. It is important to document the provenance of sources. For any sources acquired privately, keep written records. If one anticipates future public release of any sources from private collections, it is best to obtain a signed agreement up front.
Keep records about the contents of the sources for each of the variables. Preserve and document program code. Document all created variables. Keep track of key decisions made during data cleaning and preparing work file, including communications through emails, meetings, and exchanges on messaging such as Wechat.
Separating raw files and working files
Raw files contain data entered; work files are the basis for analysis. Cleaning, harmonization, and creation of variables should be a series of operations on a raw file to produce a work file.
Using data sharing agreement with collaborators
To properly share data with collaborators, students, and others, a data sharing agreement is important. It ensures a common understanding of the allowed/expected scope of analysis, collaborators’ right (if any) to keep working on the data on their own after the collaboration, if and what the collaborators can share the data further, measures to be taken to secure the data, and protocol for co-authorship and acknowledgments.
These are only some of the advises from Prof. Campbell. The seminar was held on October 8, 2020. The recording and the slides are accessible by HKUST members.
More information about Prof. Campbell’s research and datasets:
Views: 975
Go Back to page Top
- Category:
- Digital Humanities
Tags: Cameron Campbell, DH, digital humanities, digital scholarship, historical data, HKUST Research, research data management
published October 14, 2020
last modified December 28, 2022